Get Insights On Data and Data Quality
Use the Data Quality and Insights Report to perform an analysis of the data that you've imported into Data Wrangler. We recommend that you create the report after you import your dataset. You can use the report to help you clean and process your data. It gives you information such as the number of missing values and the number of outliers. If you have issues with your data, such as target leakage or imbalance, the insights report can bring those issues to your attention.
Use the following procedure to create a Data Quality and Insights report. It assumes that you've already imported a dataset into your Data Wrangler flow.
To create a Data Quality and Insights report
-
Choose a + next to a node in your Data Wrangler flow.
-
Select Get data insights.
-
For Analysis name, specify a name for the insights report.
-
(Optional) For Target column, specify the target column.
-
For Problem type, specify Regression or Classification.
-
For Data size, specify one of the following:
-
50 K – Uses the first 50000 rows of the dataset that you've imported to create the report.
-
Entire dataset – Uses the entire dataset that you've imported to create the report.
Note
Creating a Data Quality and Insights report on the entire dataset uses an Amazon SageMaker processing job. A SageMaker Processing job provisions the additional compute resources required to get insights for all of your data. For more information about SageMaker Processing jobs, see Data transformation workloads with SageMaker Processing.
-
-
Choose Create.
The following topics show the sections of the report:
You can either download the report or view it online. To download the report, choose the download button at the top right corner of the screen. The following image shows the button.

Summary
The insights report has a brief summary of the data that includes general information such as missing values, invalid values, feature types, outlier counts, and more. It can also include high severity warnings that point to probable issues with the data. We recommend that you investigate the warnings.
The following is an example of a report summary.

Target column
When you create the data quality and insights report, Data Wrangler gives you the option to select a target column. A target column is a column that you're trying to predict. When you choose a target column, Data Wrangler automatically creates a target column analysis. It also ranks the features in the order of their predictive power. When you select a target column, you must specify whether you’re trying to solve a regression or a classification problem.
For classification, Data Wrangler shows a table and a histogram of the most common classes. A class is a category. It also presents observations, or rows, with a missing or invalid target value.
The following image shows an example target column analysis for a classification problem.

For regression, Data Wrangler shows a histogram of all the values in the target column. It also presents observations, or rows, with a missing, invalid, or outlier target value.
The following image shows an example target column analysis for a regression problem.
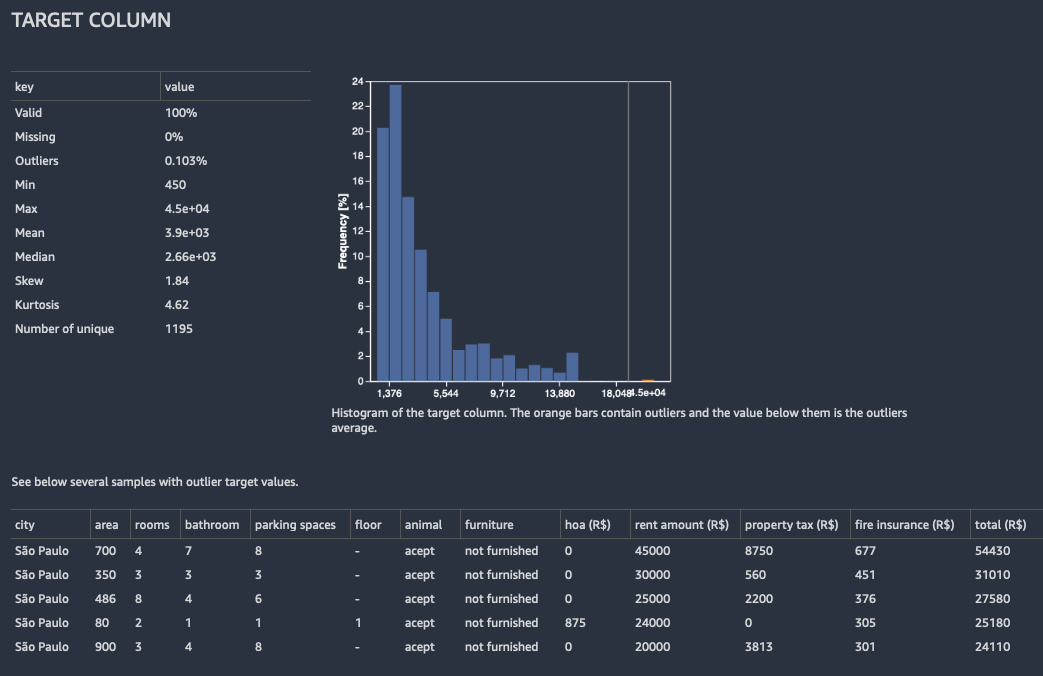
Quick model
The Quick model provides an estimate of the expected predicted quality of a model that you train on your data.
Data Wrangler splits your data into training and validation folds. It uses 80% of the samples for training and 20% of the values for validation. For classification, the sample is stratified split. For a stratified split, each data partition has the same ratio of labels. For classification problems, it's important to have the same ratio of labels between the training and classification folds. Data Wrangler trains the XGBoost model with the default hyperparameters. It applies early stopping on the validation data and performs minimal feature preprocessing.
For classification models, Data Wrangler returns both a model summary and a confusion matrix.
The following is an example of a classification model summary. To learn more about the information that it returns, see Definitions.

The following is an example of a confusion matrix that the quick model returns.

A confusion matrix gives you the following information:
-
The number of times the predicted label matches the true label.
-
The number of times the predicted label doesn't match the true label.
The true label represents an actual observation in your data. For example, if you're using a model to detect fraudulent transactions, the true label represents a transaction that is actually fraudulent or non-fraudulent. The predicted label represents the label that your model assigns to the data.
You can use the confusion matrix to see how well the model predicts the presence or the absence of a condition. If you're predicting fraudulent transactions, you can use the confusion matrix to get a sense of both the sensitivity and the specificity of the model. The sensitivity refers to the model's ability to detect fraudulent transactions. The specificity refers to the model's ability to avoid detecting non-fraudulent transactions as fraudulent.
The following is an example of the quick model outputs for a regression problem.

Feature summary
When you specify a target column, Data Wrangler orders the features by their prediction power. Prediction power is measured on the data after it was split into 80% training and 20% validation folds. Data Wrangler fits a model for each feature separately on the training fold. It applies minimal feature preprocessing and measures prediction performance on the validation data.
It normalizes the scores to the range [0,1]. Higher prediction scores indicate columns that are more useful for predicting the target on their own. Lower scores point to columns that aren’t predictive of the target column.
It’s uncommon for a column that isn’t predictive on its own to be predictive when it’s used in tandem with other columns. You can confidently use the prediction scores to determine whether a feature in your dataset is predictive.
A low score usually indicates the feature is redundant. A score of 1 implies perfect predictive abilities, which often indicates target leakage. Target leakage usually happens when the dataset contains a column that isn’t available at the prediction time. For example, it could be a duplicate of the target column.
The following are examples of the table and the histogram that show the prediction value of each feature.
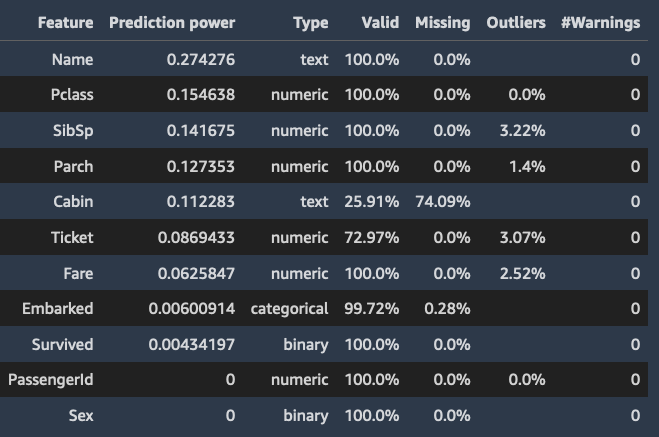

Samples
Data Wrangler provides information about whether your samples are anomalous or if there are duplicates in your dataset.
Data Wrangler detects anomalous samples using the isolation forest algorithm. The isolation forest associates an anomaly score with each sample (row) of the dataset. Low anomaly scores indicate anomalous samples. High scores are associated with non-anomalous samples. Samples with a negative anomaly score are usually considered anomalous and samples with positive anomaly score are considered non-anomalous.
When you look at a sample that might be anomalous, we recommend that you pay attention to unusual values. For example, you might have anomalous values that result from errors in gathering and processing the data. The following is an example of the most anomalous samples according to the Data Wrangler’s implementation of the isolation forest algorithm. We recommend using domain knowledge and business logic when you examine the anomalous samples.
Data Wrangler detects duplicate rows and calculates the ratio of duplicate rows in your data. Some data sources could include valid duplicates. Other data sources could have duplicates that point to problems in data collection. Duplicate samples that result from faulty data collection could interfere with machine learning processes that rely on splitting the data into independent training and validation folds.
The following are elements of the insights report that can be impacted by duplicated samples:
-
Quick model
-
Prediction power estimation
-
Automatic hyperparameter tuning
You can remove duplicate samples from the dataset using the Drop duplicates transform under Manage rows. Data Wrangler shows you the most frequently duplicated rows.
Definitions
The following are definitions for the technical terms that are used in the data insights report.