翻訳は機械翻訳により提供されています。提供された翻訳内容と英語版の間で齟齬、不一致または矛盾がある場合、英語版が優先します。
モデルバージョンを登録する
Amazon SageMaker モデルを登録するには、そのモデルが属するモデルグループを指定するモデルバージョンを作成します。モデルバージョンには、モデルのアーティファクト (モデルの学習済みの重み) とモデルの推論コードの両方が含まれている必要があります。
推論パイプラインは、推論リクエストを処理する 2~15 個のコンテナの線形シーケンスで構成される SageMaker モデルです。コンテナと関連する環境変数を指定して、推論パイプラインを登録します。推論パイプラインの詳細については、「1 つのエンドポイントの背後にあるシリアル推論パイプラインとして前処理ロジックとともにモデルをホストする」を参照してください。
コンテナと関連する環境変数を指定して、推論パイプラインを持つモデルを登録します。、 AWS SDK for Python (Boto3) Amazon SageMaker Studio コンソール、またはモデル構築パイプラインでステップを作成して、推論パイプラインで SageMaker モデルバージョンを作成するには、次のステップを使用します。
トピック
モデルバージョンを登録する (SageMakerパイプライン)
モデル構築パイプラインを使用して SageMaker モデルバージョンを登録するには、パイプラインにRegisterModel
ステップを作成します。パイプラインの一部として RegisterModel
を作成する方法の詳細については、「ステップ 8: モデルパッケージを作成する RegisterModel ステップを定義する」を参照してください。
モデルバージョンを登録する (Boto3)
Boto3 を使用してモデルバージョンを登録するには、 create_model_package
APIオペレーションを呼び出します。
まず、 create_model_package
API オペレーションに渡すようにパラメータディクショナリを設定します。
# Specify the model source model_url = "s3://
your-bucket-name/model.tar.gz
" modelpackage_inference_specification = { "InferenceSpecification": { "Containers": [ { "Image":image_uri
, "ModelDataUrl":model_url
} ], "SupportedContentTypes": [ "text/csv" ], "SupportedResponseMIMETypes": [ "text/csv" ], } } # Alternatively, you can specify the model source like this: # modelpackage_inference_specification["InferenceSpecification"]["Containers"][0]["ModelDataUrl"]=model_url create_model_package_input_dict = { "ModelPackageGroupName" : model_package_group_name, "ModelPackageDescription" : "Model to detect 3 different types of irises (Setosa, Versicolour, and Virginica)", "ModelApprovalStatus" : "PendingManualApproval" } create_model_package_input_dict.update(modelpackage_inference_specification)
次に、 create_model_package
APIオペレーションを呼び出し、先ほど設定したパラメータディクショナリを渡します。
create_model_package_response = sm_client.create_model_package(**create_model_package_input_dict) model_package_arn = create_model_package_response["ModelPackageArn"] print('ModelPackage Version ARN : {}'.format(model_package_arn))
モデルバージョンを登録する (Studio または Studio Classic)
Amazon SageMaker Studio コンソールでモデルバージョンを登録するには、Studio と Studio Classic のどちらを使用するかに基づいて、次のステップを実行します。
別のアカウントからモデルバージョンを登録する
別の AWS アカウントによって作成された Model Group にモデルバージョンを登録するには、クロスアカウント AWS Identity and Access Management リソースポリシーを追加して、そのアカウントを有効にする必要があります。例えば、組織内の 1 つの AWS アカウントがモデルのトレーニングを担当し、別のアカウントがモデルの管理、デプロイ、更新を担当します。IAM リソースポリシーを作成し、このケースへのアクセスを許可する特定のアカウントリソースにポリシーを適用します。のクロスアカウントリソースポリシーの詳細については AWS、「 ユーザーガイド」の「クロスアカウントポリシーの評価ロジックAWS Identity and Access Management 」を参照してください。
注記
また、クロスアカウントモデルデプロイのトレーニング中に、 KMSキーを使用して出力データ設定アクションを暗号化する必要があります。
でクロスアカウントモデルレジストリを有効にするには SageMaker、モデルバージョンを含むモデルグループにクロスアカウントリソースポリシーを指定する必要があります。モデルパッケージグループのクロスアカウントポリシーを作成し、その特定のリソースにこのポリシーを適用する例を以下に示します。
アカウントをまたいでモデルグループにモデルを登録するソースアカウントで、次を設定する必要があります。この例では、ソースアカウントはモデルトレーニングアカウントであり、モデルクロスアカウントをトレーニングし、Model Registry アカウントの Model Registry に登録します。
この例では、次の変数が事前に定義されていることを前提としています。
-
sm_client
– SageMaker Boto3 クライアント。 -
model_package_group_name
– アクセスを許可するモデルグループ。 -
model_package_group_arn
– クロスアカウントアクセスARNを許可するモデルグループ。 -
bucket
– モデルトレーニングアーティファクトが保存されている Amazon S3 バケット。
別のアカウントで作成されたモデルをデプロイできるようにするには、 AmazonSageMakerFullAccess
マネージドポリシーを持つロールなどの SageMaker アクションにアクセスできるロールがユーザーに必要です。 SageMaker 管理ポリシーの詳細については、「」を参照してくださいAWS Amazon の マネージドポリシー SageMaker。
必要なIAMリソースポリシー
以下の図は、クロスアカウントモデル登録を可能にするために必要なポリシーをまとめたものです。図のように、モデルを Model Registry アカウントに正しく登録するには、モデルトレーニング中これらのポリシーをに有効にする必要があります。
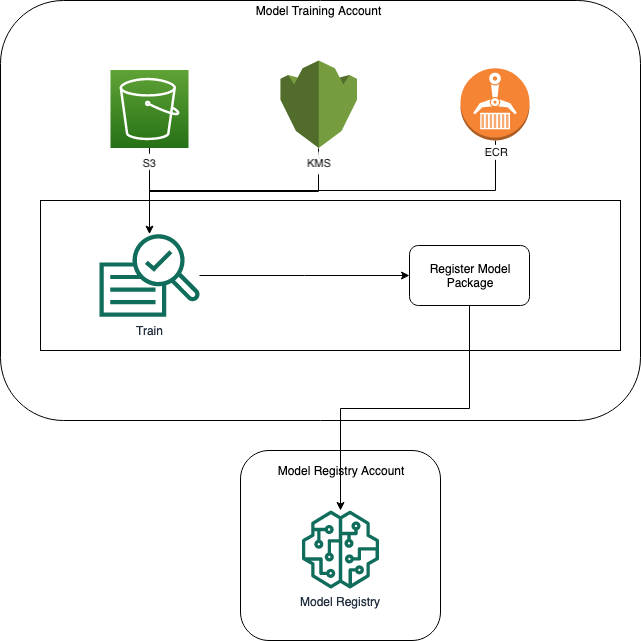
Amazon ECR、Amazon S3、および AWS KMS ポリシーは、次のコードサンプルで示されています。
Amazon ECRポリシーの例
{ "Version": "2012-10-17", "Statement": [ { "Sid": "AddPerm", "Effect": "Allow", "Principal": { "AWS": "arn:aws:iam::{
model_registry_account
}:root" }, "Action": [ "ecr:BatchGetImage", "ecr:Describe*" ] } ] }
Amazon S3 ポリシーの例
{ "Version": "2012-10-17", "Statement": [ { "Sid": "AddPerm", "Effect": "Allow", "Principal": { "AWS": "arn:aws:iam::{
model_registry_account
}:root" }, "Action": [ "s3:GetObject", "s3:GetBucketAcl", "s3:GetObjectAcl" ], "Resource": "arn:aws:s3:::{bucket
}/*" } ] }
サンプル AWS KMS ポリシー
{ "Version": "2012-10-17", "Statement": [ { "Sid": "AddPerm", "Effect": "Allow", "Principal": { "AWS": "arn:aws:iam::{
model_registry_account
}:root" }, "Action": [ "kms:Decrypt", "kms:GenerateDataKey*" ], "Resource": "*" } ] }
リソースポリシーをアカウントに適用
以下のポリシー設定は、前のセクションで説明したポリシーを適用し、モデルトレーニングアカウントに含める必要があります。
import json # The Model Registry account id of the Model Group model_registry_account = "
111111111111
" # The model training account id where training happens model_training_account = "222222222222
" # 1. Create a policy for access to the ECR repository # in the model training account for the Model Registry account Model Group ecr_repository_policy = {"Version": "2012-10-17", "Statement": [{"Sid": "AddPerm", "Effect": "Allow", "Principal": { "AWS": f"arn:aws:iam::{model_registry_account}:root" }, "Action": [ "ecr:BatchGetImage", "ecr:Describe*" ] }] } # Convert the ECR policy from JSON dict to string ecr_repository_policy = json.dumps(ecr_repository_policy) # Set the new ECR policy ecr = boto3.client('ecr') response = ecr.set_repository_policy( registryId = model_training_account, repositoryName = "decision-trees-sample", policyText = ecr_repository_policy ) # 2. Create a policy in the model training account for access to the S3 bucket # where the model is present in the Model Registry account Model Group bucket_policy = {"Version": "2012-10-17", "Statement": [{"Sid": "AddPerm", "Effect": "Allow", "Principal": {"AWS": f"arn:aws:iam::{model_registry_account}:root" }, "Action": [ "s3:GetObject", "s3:GetBucketAcl", "s3:GetObjectAcl" ], "Resource": [ "arn:aws:s3:::{bucket
}/*", "Resource: arn:aws:s3:::{bucket
}" ] }] } # Convert the S3 policy from JSON dict to string bucket_policy = json.dumps(bucket_policy) # Set the new bucket policy s3 = boto3.client("s3") response = s3.put_bucket_policy( Bucket =bucket
, Policy = bucket_policy) # 3. Create the KMS grant for the key used during training for encryption # in the model training account to the Model Registry account Model Group client = boto3.client("kms") response = client.create_grant( GranteePrincipal=model_registry_account, KeyId=kms_key_id Operations=[ "Decrypt", "GenerateDataKey", ], )
次の設定は、Model Group がある Model Registry アカウントに配置する必要があります。
# The Model Registry account id of the Model Group model_registry_account = "
111111111111
" # 1. Create policy to allow the model training account to access the ModelPackageGroup model_package_group_policy = {"Version": "2012-10-17", "Statement": [ { "Sid": "AddPermModelPackageVersion", "Effect": "Allow", "Principal": {"AWS": f"arn:aws:iam::{model_training_account
}:root"}, "Action": ["sagemaker:CreateModelPackage"], "Resource": f"arn:aws:sagemaker:{region}:{model_registry_account}:model-package/{model_package_group_name
}/*" } ] } # Convert the policy from JSON dict to string model_package_group_policy = json.dumps(model_package_group_policy) # Set the new policy response = sm_client.put_model_package_group_policy( ModelPackageGroupName =model_package_group_name
, ResourcePolicy = model_package_group_policy)
最後に、モデルトレーニングアカウントの create_model_package
アクションを使用して、モデルパッケージをクロスアカウントに登録します。
# Specify the model source model_url = "s3://{
bucket
}/model.tar.gz" #Set up the parameter dictionary to pass to the create_model_package API operation modelpackage_inference_specification = { "InferenceSpecification": { "Containers": [ { "Image": f"{model_training_account
}.dkr.ecr.us-east-2.amazonaws.com/decision-trees-sample:latest", "ModelDataUrl": model_url } ], "SupportedContentTypes": [ "text/csv" ], "SupportedResponseMIMETypes": [ "text/csv" ], } } # Alternatively, you can specify the model source like this: # modelpackage_inference_specification["InferenceSpecification"]["Containers"][0]["ModelDataUrl"]=model_url create_model_package_input_dict = { "ModelPackageGroupName" :model_package_group_arn
, "ModelPackageDescription" : "Model to detect 3 different types of irises (Setosa, Versicolour, and Virginica)", "ModelApprovalStatus" : "PendingManualApproval" } create_model_package_input_dict.update(modelpackage_inference_specification) # Create the model package in the Model Registry account create_model_package_response = sm_client.create_model_package(**create_model_package_input_dict) model_package_arn = create_model_package_response["ModelPackageArn"] print('ModelPackage Version ARN : {}'.format(model_package_arn))