파이프라인 단계의 선택적 실행
Pipelines을 사용하여 워크플로를 만들고 ML 훈련 단계를 조율할 때 여러 실험 단계를 수행해야 할 수 있습니다. 매번 전체 파이프라인을 실행하는 대신 특정 단계만 반복하면 됩니다. Pipelines을 사용하면 파이프라인 단계를 선택적으로 실행할 수 있습니다. 이렇게 하면 ML 훈련을 최적화하는 데 도움이 됩니다. 선택적 실행은 다음 시나리오에서 유용합니다.
업스트림 단계의 파라미터를 유지하면서 업데이트된 인스턴스 유형, 하이퍼파라미터 또는 기타 변수를 사용하여 특정 단계를 다시 시작하려고 합니다.
파이프라인이 중간 단계에서 실패합니다. 데이터 준비 또는 특징 추출과 같은 이전 실행 단계는 다시 실행하는 데 비용이 많이 듭니다. 파이프라인을 완료하려면 수정 사항을 적용하고 특정 단계를 수동으로 다시 실행해야 할 수 있습니다.
선택적 실행을 사용하면 파이프라인의 방향성 비순환 그래프(DAG)에 연결되어 있는 한 일부 단계를 실행하도록 선택할 수 있습니다. 다음 DAG는 예제 파이프라인 워크플로를 보여줍니다.
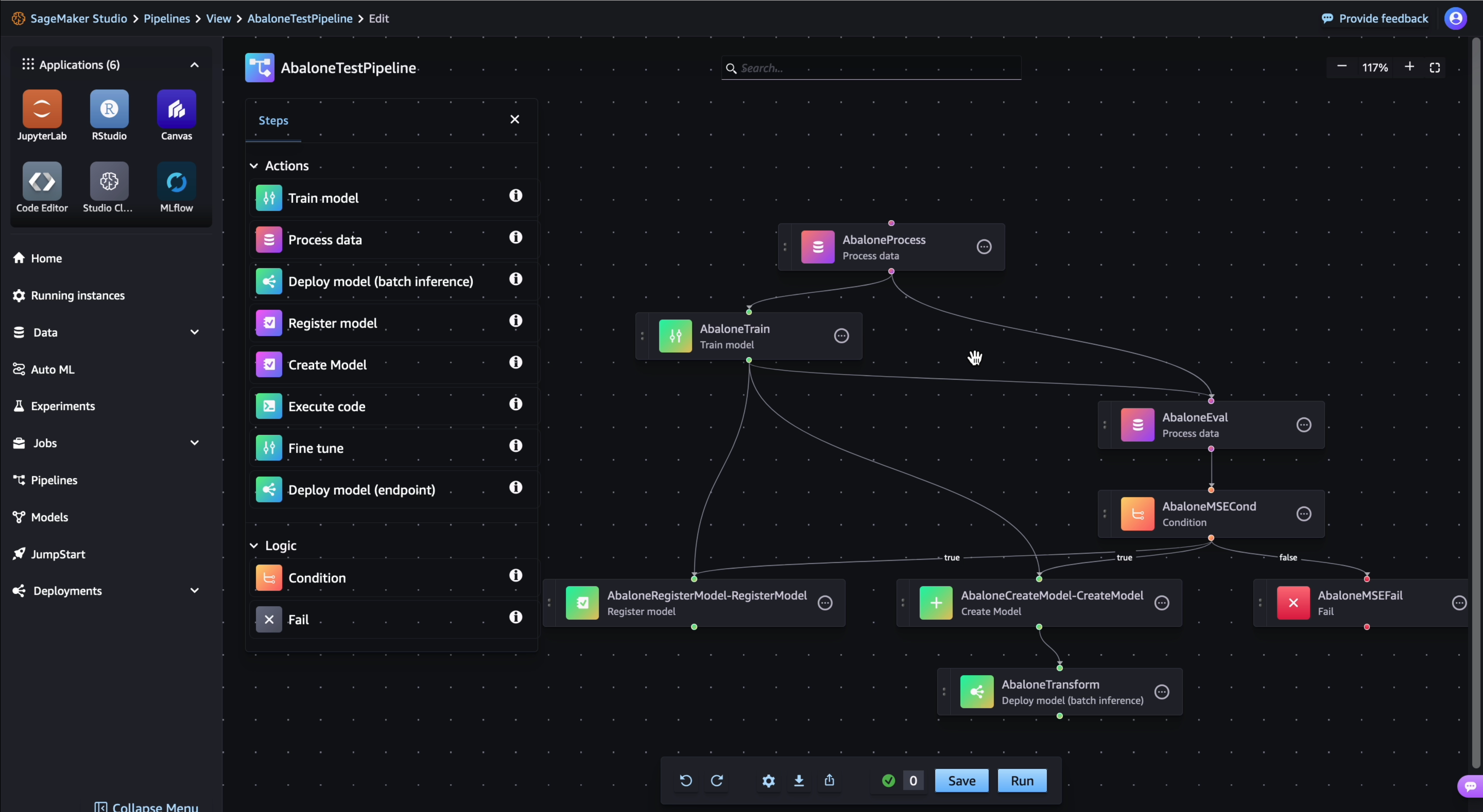
선택적 실행에서는 AbaloneTrain
단계와 AbaloneEval
단계를 선택할 수 있지만 DAG에서 AbaloneTrain
, AbaloneMSECond
단계가 연결되어 있지 않기 때문에 이 단계만 선택할 수는 없습니다. 워크플로에서 선택되지 않은 단계의 경우 선택적 실행은 단계를 다시 실행하는 대신 참조 파이프라인 실행의 출력을 재사용합니다. 또한 선택한 단계의 다운스트림에 있는 선택되지 않은 단계는 선택적 실행에서 실행되지 않습니다.
파이프라인에서 중간 단계 중 일부를 실행하도록 선택하면 단계가 이전 단계에 종속될 수 있습니다. SageMaker는 이러한 종속성을 리소싱할 참조 파이프라인 실행이 필요합니다. 예를 들어, AbaloneTrain
및 AbaloneEval
단계를 실행하도록 선택한 경우 AbaloneProcess
단계에 대한 출력이 필요합니다. 참조 실행 ARN을 제공하거나 SageMaker가 기본 동작인 최신 파이프라인 실행을 사용하도록 지시할 수 있습니다. 참조 실행이 있는 경우 참조 실행에서 런타임 파라미터를 빌드하여 재정의와 함께 선택적 실행에 제공할 수도 있습니다. 세부 정보는 참조 실행의 런타임 파라미터 값을 재사용합니다.을 참조하세요.
세부 사항으로는 SelectiveExecutionConfig
를 사용하여 선택적 실행 파이프라인 실행을 위한 구성을 제공합니다. 참조 파이프라인 실행(source_pipeline_execution_arn
인수 포함)에 대해 ARN을 포함하는 경우 SageMaker는 제공한 파이프라인 실행의 이전 단계 종속성을 사용합니다. ARN을 포함하지 않고 최신 파이프라인 실행이 존재하는 경우 SageMaker는 기본적으로 최신 파이프라인 실행을 참조로 사용합니다. ARN을 포함하지 않고 SageMaker가 최신 파이프라인 실행을 사용하지 않도록 하려면 reference_latest_execution
을 False
로 설정하세요. SageMaker가 최종적으로 참조로 사용하는 파이프라인 실행(최신 또는 사용자 지정 파이프라인)은 Success
또는 Failed
상태여야 합니다.
다음 표에는 SageMaker가 참조 실행을 선택하는 방법이 요약되어 있습니다.
source_pipeline_execution_arn 인수 값 |
reference_latest_execution 인수 값 |
사용된 참조 실행 |
---|---|---|
파이프라인 ARN |
|
지정된 파이프라인 ARN |
파이프라인 ARN |
|
지정된 파이프라인 ARN |
null 또는 지정되지 않음 |
|
최신 파이프라인 실행 |
null 또는 지정되지 않음 |
|
없음 - 이 경우 업스트림 종속성이 없는 단계를 선택하세요. |
선택적 실행 구성 요구 사항에 대한 자세한 내용은 Sagemaker.workflow.Selective_Execution_config.SelectiveExecutionConfig
다음 설명에는 파이프라인 참조 실행을 지정하거나, 최신 파이프라인 실행을 참조로 사용하거나, 참조 파이프라인 실행 없이 선택적 실행을 실행하려는 경우의 예제가 포함되어 있습니다.
사용자 지정 파이프라인 참조를 사용한 선택적 실행
다음 예시는 참조 파이프라인 실행을 사용한 AbaloneTrain
단계와 AbaloneEval
단계의 선택적 실행을 보여줍니다.
from sagemaker.workflow.selective_execution_config import SelectiveExecutionConfig selective_execution_config = SelectiveExecutionConfig( source_pipeline_execution_arn="arn:aws:sagemaker:us-west-2:123123123123:pipeline/abalone/execution/123ab12cd3ef", selected_steps=["AbaloneTrain", "AbaloneEval"] ) selective_execution = pipeline.start( execution_display_name=f"Sample-Selective-Execution-1", parameters={"MaxDepth":6, "NumRound":60}, selective_execution_config=selective_execution_config, )
최신 파이프라인 실행을 참조로 사용한 선택적 실행
다음 예시는 최신 파이프라인 실행을 참조로 사용한 AbaloneTrain
단계와 AbaloneEval
단계의 선택적 실행을 보여줍니다. SageMaker는 기본적으로 최신 파이프라인 실행을 사용하므로 선택적으로 reference_latest_execution
인수를 True
로 설정할 수 있습니다.
# Prepare a new selective execution. Select only the first step in the pipeline without providing source_pipeline_execution_arn. selective_execution_config = SelectiveExecutionConfig( selected_steps=["AbaloneTrain", "AbaloneEval"], # optional reference_latest_execution=True ) # Start pipeline execution without source_pipeline_execution_arn pipeline.start( execution_display_name=f"Sample-Selective-Execution-1", parameters={"MaxDepth":6, "NumRound":60}, selective_execution_config=selective_execution_config, )
참조 파이프라인 없이 선택적 실행
다음 예시는 참조 ARN을 제공하지 않고 최신 파이프라인 실행을 참조로 사용하는 옵션을 끈 상태에서 AbaloneProcess
단계와 AbaloneTrain
단계의 선택적 실행을 보여줍니다. SageMaker는 이 단계 하위 집합이 이전 단계에 종속되지 않으므로 이 구성을 허용합니다.
# Prepare a new selective execution. Select only the first step in the pipeline without providing source_pipeline_execution_arn. selective_execution_config = SelectiveExecutionConfig( selected_steps=["AbaloneProcess", "AbaloneTrain"], reference_latest_execution=False ) # Start pipeline execution without source_pipeline_execution_arn pipeline.start( execution_display_name=f"Sample-Selective-Execution-1", parameters={"MaxDepth":6, "NumRound":60}, selective_execution_config=selective_execution_config, )
참조 실행의 런타임 파라미터 값을 재사용합니다.
build_parameters_from_execution
을 사용하여 참조 파이프라인 실행에서 파라미터를 빌드하고 선택적 실행 파이프라인에 결과를 제공할 수 있습니다. 참조 실행의 원래 파라미터를 사용하거나 parameter_value_overrides
인수를 사용하여 재정의를 적용할 수 있습니다.
다음 예제는 참조 실행에서 파라미터를 빌드하고 MseThreshold
파라미터에 대한 재정의를 적용하는 방법을 보여줍니다.
# Prepare a new selective execution. selective_execution_config = SelectiveExecutionConfig( source_pipeline_execution_arn="arn:aws:sagemaker:us-west-2:123123123123:pipeline/abalone/execution/123ab12cd3ef", selected_steps=["AbaloneTrain", "AbaloneEval", "AbaloneMSECond"], ) # Define a new parameters list to test. new_parameters_mse={ "MseThreshold": 5, } # Build parameters from reference execution and override with new parameters to test. new_parameters = pipeline.build_parameters_from_execution( pipeline_execution_arn="arn:aws:sagemaker:us-west-2:123123123123:pipeline/abalone/execution/123ab12cd3ef", parameter_value_overrides=new_parameters_mse ) # Start pipeline execution with new parameters. execution = pipeline.start( selective_execution_config=selective_execution_config, parameters=new_parameters )