本文為英文版的機器翻譯版本,如內容有任何歧義或不一致之處,概以英文版為準。
設定偵錯工具規則的筆記本和程式碼範例範例
在以下各節中,會提供筆記本和程式碼範例,說明如何使用 Debugger 規則來監控 SageMaker 訓練任務。
偵錯工具內建規則範例筆記本
下列範例筆記本示範如何在使用 Amazon 執行訓練工作時使用 Debugger 內建規則 SageMaker:
在 SageMaker Studio 中執行範例筆記本時,您可以在 Studio 實驗清單索引標籤上找到建立的訓練任務試用版。例如,如下列螢幕擷取畫面所示,您可以尋找並開啟目前訓練任務的描述試驗元件視窗。在偵錯工具索引標籤上,您可以檢查偵錯程式規則 (vanishing_gradient()
和 loss_not_decreasing()
) 是否平行監控訓練任務工作階段。如需如何在 Studio UI 中尋找訓練任務試用元件的完整說明,請參閱 SageMaker Studio - 檢視實驗、試用和試用元件。
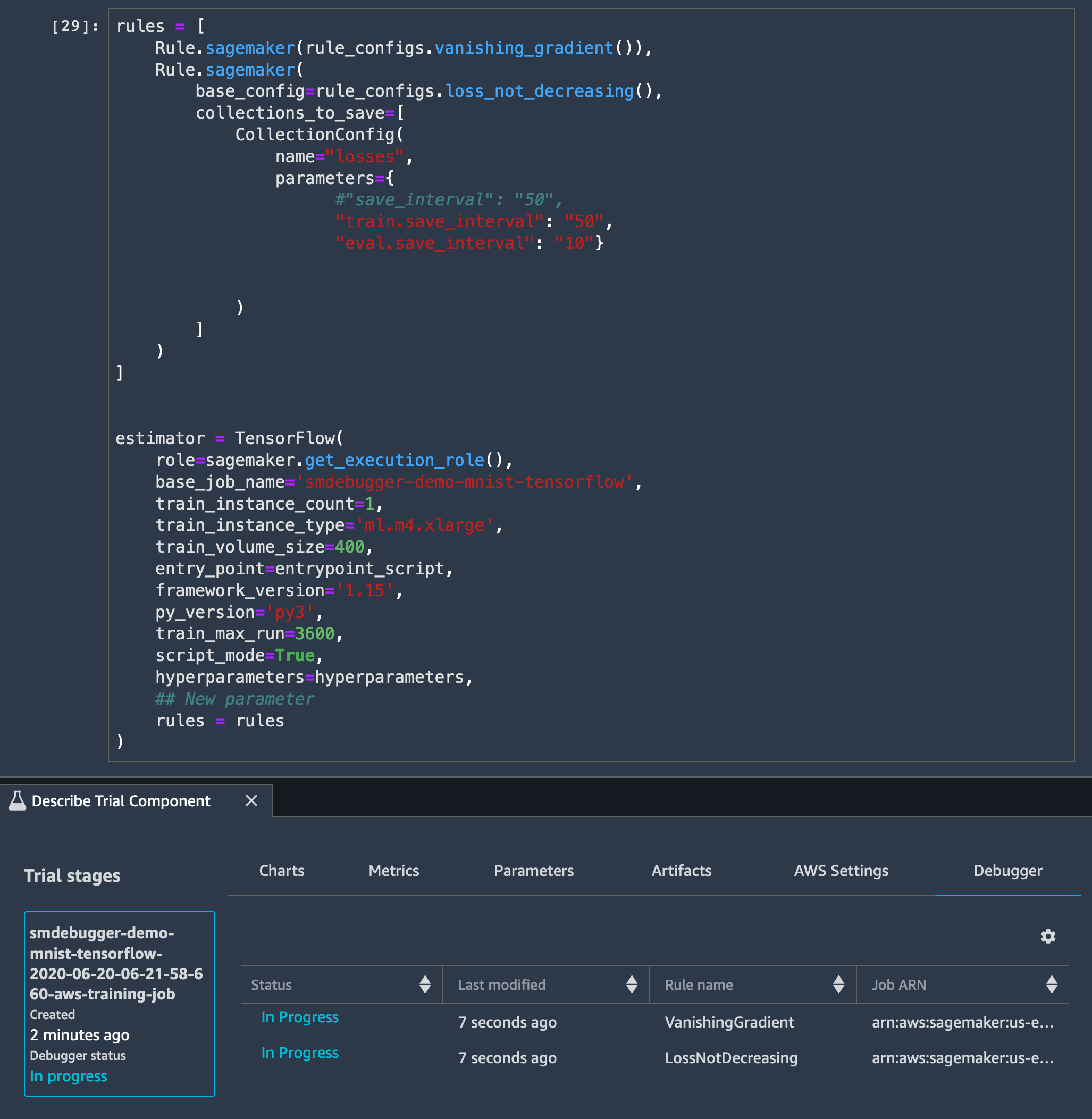
有兩種方法可以在 SageMaker 環境中使用 Debugger 內建規則:在準備內建規則時部署規則,或視需要調整其參數。下列主題示範如何搭配範例程式碼使用內建規則。
偵錯工具內建規則範例程式碼
下列程式碼範例示範如何使用 Rule.sagemaker
方法設定偵錯工具內建規則。若要指定您要執行的內建規則,請使用 rules_configs
API操作來呼叫內建規則。要查找 Debugger 內建規則和預設參數值的完整清單,請參閱Debugger 內建規則清單。
import sagemaker from sagemaker.tensorflow import TensorFlow from sagemaker.debugger import Rule, CollectionConfig, rule_configs # call built-in rules that you want to use. built_in_rules=[ Rule.sagemaker(rule_configs.vanishing_gradient()) Rule.sagemaker(rule_configs.loss_not_decreasing()) ] # construct a SageMaker estimator with the Debugger built-in rules sagemaker_estimator=TensorFlow( entry_point='directory/to/your_training_script.py', role=sm.get_execution_role(), base_job_name='debugger-built-in-rules-demo', instance_count=1, instance_type="
ml.p3.2xlarge
", framework_version="2.9.0
", py_version="py39
", # debugger-specific arguments below rules=built_in_rules ) sagemaker_estimator.fit()
注意
偵錯工具內建規則會與您的訓練任務平行執行。訓練任務的內建規則容器數量上限為 20。
如需偵錯工具規則類別、方法和參數的詳細資訊,請參閱 Amazon SageMaker Python SDK
要查找有關如何調整 Debugger 規則參數的範例,請參閱以下 透過參數修改使用 Debugger 內建規則 部分。
透過參數修改使用 Debugger 內建規則
下列程式碼範例示範調整參數的內建規則結構。在此範例中,stalled_training_rule
會每 50 個步驟會從訓練任務收集 losses
張量集合,並每 10 個步驟收集評估階段。如果訓練程序開始停止,而且在 120 秒內未收集張量輸出,則 stalled_training_rule
會停止訓練任務。
import sagemaker from sagemaker.tensorflow import TensorFlow from sagemaker.debugger import Rule, CollectionConfig, rule_configs # call the built-in rules and modify the CollectionConfig parameters base_job_name_prefix= 'smdebug-stalled-demo-' + str(int(time.time())) built_in_rules_modified=[ Rule.sagemaker( base_config=rule_configs.stalled_training_rule(), rule_parameters={ 'threshold': '120', 'training_job_name_prefix': base_job_name_prefix, 'stop_training_on_fire' : 'True' } collections_to_save=[ CollectionConfig( name="losses", parameters={ "train.save_interval": "50" "eval.save_interval": "10" } ) ] ) ] # construct a SageMaker estimator with the modified Debugger built-in rule sagemaker_estimator=TensorFlow( entry_point='directory/to/your_training_script.py', role=sm.get_execution_role(), base_job_name=base_job_name_prefix, instance_count=1, instance_type="
ml.p3.2xlarge
", framework_version="2.9.0
", py_version="py39
", # debugger-specific arguments below rules=built_in_rules_modified ) sagemaker_estimator.fit()