本文為英文版的機器翻譯版本,如內容有任何歧義或不一致之處,概以英文版為準。
啟用檢查點後,SageMaker AI 會將檢查點儲存到 Amazon S3,並使用檢查點 S3 儲存貯體同步您的訓練任務。您可以針對檢查點 S3 儲存貯體使用 S3 一般用途或 S3 目錄儲存貯體。
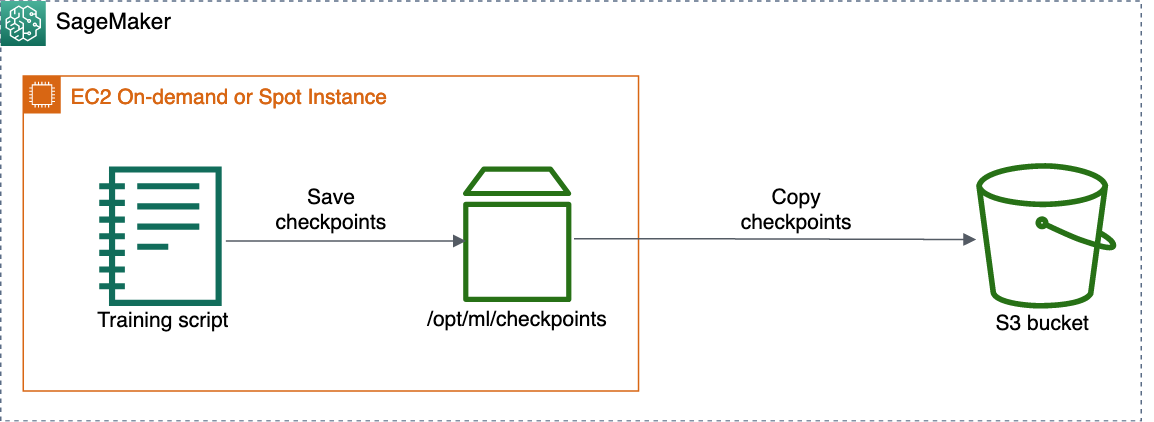
下列範例示範如何在建構 SageMaker AI 估算器時設定檢查點路徑。若要啟用檢查點,請將 checkpoint_s3_uri
和 checkpoint_local_path
參數新增至您的估算器。
下列範例範本示範如何建立一般 SageMaker AI 估算器並啟用檢查點。您可以指定 image_uri
參數,使用適用於支援之演算法的範本。若要尋找具有 SageMaker AI 支援檢查點之演算法的 Docker 映像 URIs,請參閱 Docker 登錄檔路徑和範例程式碼。您也可以將 estimator
和 取代Estimator
為其他 SageMaker AI 架構的估算器父類別和估算器類別,例如 TensorFlow
、PyTorch
、 MXNet
HuggingFace
和 XGBoost
。
import sagemaker
from sagemaker.estimator
import Estimator
bucket=sagemaker.Session().default_bucket()
base_job_name="sagemaker-checkpoint-test
"
checkpoint_in_bucket="checkpoints
"
# The S3 URI to store the checkpoints
checkpoint_s3_bucket="s3://{}/{}/{}".format(bucket, base_job_name, checkpoint_in_bucket)
# The local path where the model will save its checkpoints in the training container
checkpoint_local_path="/opt/ml/checkpoints"
estimator = Estimator
(
...
image_uri="<ecr_path>
/<algorithm-name>
:<tag>
" # Specify to use built-in algorithms
output_path=bucket,
base_job_name=base_job_name,
# Parameters required to enable checkpointing
checkpoint_s3_uri=checkpoint_s3_bucket,
checkpoint_local_path=checkpoint_local_path
)
下列兩個參數會指定檢查點的路徑:
-
checkpoint_local_path
— 指定模型在訓練容器中定期儲存檢查點的本機路徑。預設路徑設定為'/opt/ml/checkpoints'
。如果您正在使用其他架構或使用自己的訓練容器,請確定訓練指令碼的檢查點組態已指定路徑為'/opt/ml/checkpoints'
。注意
我們建議指定本機路徑
'/opt/ml/checkpoints'
,以符合預設的 SageMaker AI 檢查點設定。如果您偏好指定自己的本機路徑,請務必比對訓練指令碼中的檢查點儲存路徑和 SageMaker AI 估算器的checkpoint_local_path
參數。 -
checkpoint_s3_uri
— URI 導向即時儲存檢查點的 S3 儲存貯體。您可以指定 S3 一般用途或 S3 目錄儲存貯體來存放檢查點。如需 S3 目錄儲存貯體的詳細資訊,請參閱《Amazon Simple Storage Service 使用者指南》中的目錄儲存貯體。
若要尋找 SageMaker AI 估算器參數的完整清單,請參閱 Amazon SageMaker Python SDK