기계 번역으로 제공되는 번역입니다. 제공된 번역과 원본 영어의 내용이 상충하는 경우에는 영어 버전이 우선합니다.
SageMaker AI 모델 섀도우 배포를 사용하여 장기 실행 섀도우 변형을 생성하여 모델 제공 스택의 새 후보 구성 요소를 검증한 후 프로덕션으로 승격할 수 있습니다. 다음 다이어그램은 섀도우 변형의 작동 방식을 자세히 보여줍니다.
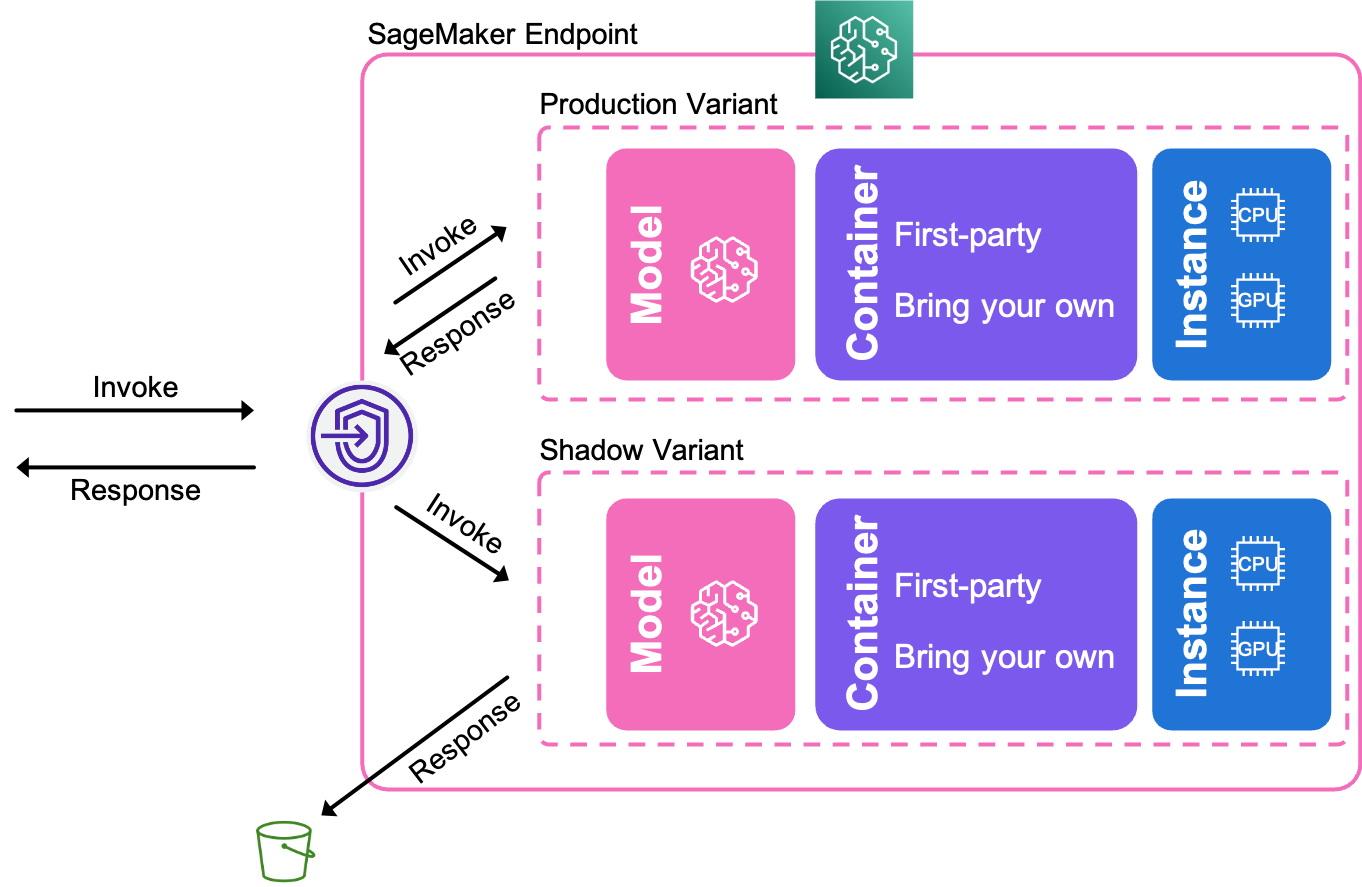
섀도우 변형 배포
다음 코드 예제는 섀도우 변형을 프로그래밍 방식으로 배포하는 방법을 보여줍니다. 예제의 사용자 자리표시자 텍스트
를 본인의 정보로 대체하세요.
-
두 개의 SageMaker AI 모델을 생성합니다. 하나는 프로덕션 변형용이고 다른 하나는 섀도우 변형용입니다.
import boto3 from sagemaker import get_execution_role, Session aws_region = "
aws-region
" boto_session = boto3.Session(region_name=aws_region) sagemaker_client = boto_session.client("sagemaker") role = get_execution_role() bucket = Session(boto_session).default_bucket() model_name1 = "name-of-your-first-model
" model_name2 = "name-of-your-second-model
" sagemaker_client.create_model( ModelName = model_name1, ExecutionRoleArn = role, Containers=[ { "Image": "ecr-image-uri-for-first-model
", "ModelDataUrl": "s3-location-of-trained-first-model
" } ] ) sagemaker_client.create_model( ModelName = model_name2, ExecutionRoleArn = role, Containers=[ { "Image": "ecr-image-uri-for-second-model
", "ModelDataUrl": "s3-location-of-trained-second-model
" } ] ) -
엔드포인트 구성 생성. 구성에서 프로덕션과 섀도우 버전을 모두 지정합니다.
endpoint_config_name =
name-of-your-endpoint-config
create_endpoint_config_response = sagemaker_client.create_endpoint_config( EndpointConfigName=endpoint_config_name, ProductionVariants=[ { "VariantName":name-of-your-production-variant
, "ModelName": model_name1, "InstanceType":"ml.m5.xlarge"
, "InitialInstanceCount":1
, "InitialVariantWeight":1
, } ], ShadowProductionVariants=[ { "VariantName":name-of-your-shadow-variant
, "ModelName": model_name2, "InstanceType":"ml.m5.xlarge"
, "InitialInstanceCount":1
, "InitialVariantWeight":1
, } ] ) -
엔드포인트를 생성합니다.
create_endpoint_response = sm.create_endpoint( EndpointName=
name-of-your-endpoint
, EndpointConfigName=endpoint_config_name, )